It’s a turning point. Medicine can be personalized and the costs of treatment can be reduced… Technology solutions may support doctors and help them to improve their efficiency, says Marcin Sieniek from Google Health in conversation with Monika Redzisz
Monika Redzisz: At the beginning of this year, “Nature” published an article you co-authored, summarizing the results of the work of researchers from Google Health and Imperial College in London on an artificial intelligence model capable of diagnosing breast cancer. The issue is being tackled by many scientific groups, including the New York University (NYU) team led by Krzysztof Geras and the MIT team. We can say that it’s a kind of race in which the researchers are trying to come up with the most efficient model. What makes your model different from others?
Marcin Sieniek*: I wouldn’t say it’s a race. It’s more like friendly competition. The teams you mentioned wrote two of my favorite articles on applying artificial intelligence in breast cancer diagnostics. But it’s true that we have adopted a slightly different approach to the matter.
What makes the team led by Krzysztof Geras exceptional is the fact that the images they work on are analyzed by a considerable number of specialists: mammograms of 720 patients have been interpreted by 14 radiologists. We could afford only 5 radiologists to study 500 cases. Although both teams limit themselves to laboratory evaluation, we have gone a step further. We are analyzing the results in order to find out to what extent the assumptions were correct. The hospitals we collaborate with have provided us not only with de-identified medical images (i.e. images containing no data making it possible to identify patients), but also with the information about what the radiologist actually read after analyzing patients’ images. After some time, the hospitals make a decision about how to proceed in individual cases: they verify which patients got sick and which did not. This allows us to say “who was right”. In clinical settings, radiologists have access to additional information that is not available in laboratories. In our opinion, the analysis of algorithms only in laboratory conditions is incomplete.
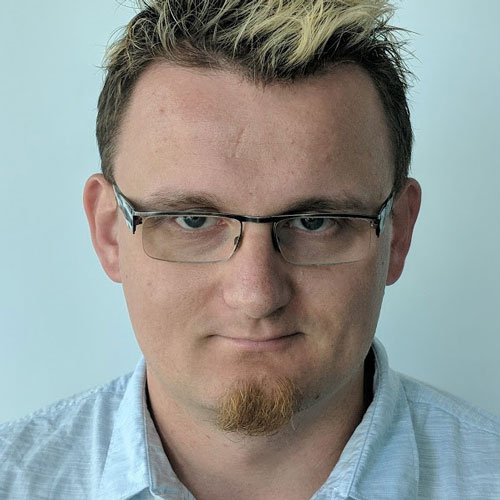
Is artificial intelligence as good as radiologists?
Yes, but in the end it doesn’t matter. What is important, however, is how the model works in practice and whether it prove useful to a doctor. In the course of their practice, radiologists have access not only to one particular mammogram, but also to mammograms and ultrasound scans from previous years and to notes from previous visits; they can even call GPs and ask them if their patients have complained of any kind of pain. They have much additional, indirect and often very subtle information. Despite all that, our model has shown that, as far as efficiency is concerned, in certain specific cases it can be as good as and sometimes even better than radiologists.
The MIT team claim that they are able to diagnose breast cancer five years before the first symptoms appear. How long could I feel safe after your examination?
It’s hard to be sure in such a long perspective. The idea is to get the information on whether the patient is at high risk or whether they should be examined more often, because most of the research in this field can be used to diagnose the disease in a fairly short-term perspective. The period of 120 days to which the team led by professor Geras refers means that the patient shows no sign of cancer today and that the disease will not develop for the next four months. However, we don’t know what will happen after that. We are taking a longer term perspective adapted to the program in a given country. In the UK it’s three years, in the USA it’s one or two years. So when we say that a patient shows no symptoms of cancer, we mean that she should be fine until the next visit (although we mustn’t forget that some types of cancer appear suddenly and are not visible on the mammograms from the previous visit – those are impossible to predict).
How many mammograms did you use to train the model?
To train the model we used tens of thousands of images. As far as the Great Britain is concerned, the test set consisted of 100 thousand images. In the case of the United States, the set was smaller but it included difficult cases. Although our set was not as impressive as NYU’s, which comprised about one million medical images, we managed to get very good results.
Did you have adequate representation of patients from different ethnic groups?
Luckily, both the USA and the UK are ethnically diverse countries. Unfortunately, we had too few patients from some ethnic groups to clearly determine that our model is better than doctors in their case. But our internal analysis did not show that the model worked worse for any of the minority groups. This issue is very important for us, so before the research, we made sure that we were following the standards of ethical AI development.
When will the technique be available to patients?
These kinds of solutions are usually implemented within three years. Typically, obtaining approvals in a single medical market usually takes 12 to 18 months. Then, you need to carry out clinical trials. The patients should be randomly divided into two groups. The first one should be diagnosed according to standard procedures and the second one should be diagnosed with the use of the tested method. After that, it will take another two years to see if the prognoses are correct. Only then will we be sure that the new technique is better than the one that has been used so far.
Naturally, before all that happens, we will be carrying out tests to which all patients will be invited. However, they should be aware that this is not yet a health standard.
Healthcare has not benefited much from the Internet revolution, although it has impacted almost every area of our lives
How did you find a job in Google Health?
I finished my studies in Kraków, I took a PhD in computer science. I’m also a bachelor in business. During my studies I founded a tech startup called “MailGrupowy.pl”. The initiative turned out to be a flop and I submitted my job application with Google. After some time I was given the opportunity to work in a different division within the company. The places I could choose from included Warsaw, Zurich and Irvine, California. California sounded like a nice play to live, I admit. Sunny weather, ocean waters, mountain ranges. What’s not to like? I decided to emigrate. After three years in Irvine I moved to northern California to work in the company’s headquarters on medical projects.
Why?
I knew what artificial intelligence had to offer and I asked myself a question: “Where can that tool be used to its fullest advantage?” The reason why screening is so interesting for artificial intelligence is the fact that it involves a large volume of relatively standardized images.
In general, there is a lot of room for innovation in medicine today. Healthcare has not benefited much from the Internet revolution, although it has impacted almost every area of our lives. We may have seen some quality medical solutions, but they have not translated into how healthcare services are provided. But now it’s a turning point. Medicine can be personalized, the costs of treatment can be reduced, and some processes can be streamlined. Technology solutions may support doctors and help them to improve their efficiency.
You also worked for Tesla…
Yes, but not for long. I got a job in the Autopilot team where we worked on artificial intelligence for self-driving vehicles. But I quickly realized that I preferred working for Google, where the organizational culture is exceptional. The company has a bottom-up structure and an engineer has a lot to say in the process of developing a project.
I’ve been always trying to do meaningful things. I don’t want to sound pretentious but I’m looking for the ways to apply artificial intelligence to make the world a better place. In my opinion, both medicine and autonomous vehicles are the fields where artificial intelligence could make a difference.
For many Americans healthcare means inequality. Will technological innovations result in fairer access to medical treatment?
I hope so. Innovations can drive down the costs and personalize treatment. This may be particularly important for the groups that have historically been treated less favorably as the standards have been developed on the basis of privileged groups. But the problem won’t disappear unless we do something to with it.
*Marcin Sieniek took his PhD in computer science from the AGH University of Science and Technology in Kraków. He also received his bachelor’s degree in management from the Cracow University of Economics. During his studies he founded a startup whose activity was co-financed by foreign capital and consisted in managing the MailGrupowy.pl social and learning website. After moving to California, he worked for the Tesla Autopilot team. He later joined Google, where he has been involved in projects focused on artificial intelligence in advertising and medicine.
Przeczytaj polską wersję tego tekstu TUTAJ